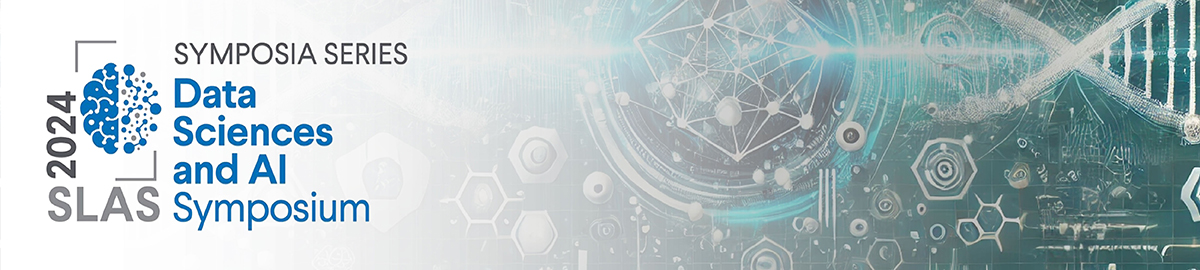
October 28, 2024
Excited or apprehensive about how data science is shaping future research? Machine learning, generative AI, image analytics, and analyzing omics data types are valuable tools. Taking the wheel to decide how and when to use these worthwhile assets requires careful study and intentional education.
While no one really believes that AI will lead to robots taking over the world, at this current moment in time the media buzz does make it feel like an uprising. How do you gain the advantage of the subject and shut out all the clamor? Indulge your curiosity, say Ian Kerman, M.Sc., and Anupama Reddy, Ph.D., the co-chairs of the upcoming SLAS 2024 Data Science and AI Symposium, to be held November 12-13, 2024, on the campus of Novartis BioMedical Research (Cambridge, MA, USA).
Kerman suggests the first step in AI 101 is to shift your mindset. He describes two diverse types of researchers who have trouble adopting AI technologies — those who are hesitant to adopt AI because they are uncertain the results are trustworthy and those who rush to use it because they don’t want to fall behind.
“If the research team decides to jump into AI without considering the problem they're trying to solve, they are almost assured of becoming frustrated or let down because the results aren't interesting or good. Then they move on, leaving AI with the stain of ‘it wasn't useful for what I do’,” Kerman says.
“Persuading skeptics of AI’s value is a difficult barrier to break. Consultants and service providers must build trust in the process,” he continues. Determining first what problem to solve “changes the focus of AI from a new and shiny technology that can do everything, to a tool that allows you to have a conversation with your data.”
Reddy agrees. “Some bench scientists come in thinking data scientists can just push a button and the answer pops out. Yes, you can push a button and an answer will pop out, but that might not be the right answer. The value of data science lies in understanding both the question and data, and in connecting the dots using appropriate methods. Just like in science and research, the answer lies in iterations to go deeper into the question/data."
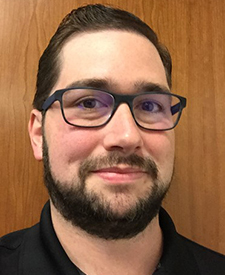
Kerman sees himself in the upcoming symposium participants, and “one thing that I've always noticed about myself is when I'm working on a project or with a client, I tend to get very tunnel-visioned and focused on solving the problem,” he observes. “Having events like the upcoming [SLAS] Data Science and AI Symposium allows me to see what others are doing in similar situations. What technologies and techniques are they using to solve problems? Very often, they are things that I haven’t considered. We might be using the same type of technology, but they're using it to solve a vastly different problem, or they're using it in quite a different way than I had thought of using it.”
Kerman’s background is rooted in molecular biology and bioinformatics. While he started his career in a biotech lab, he quickly shifted over to a software company.
“We specialized in making data pipelining software for scientists,” Kerman explains. “I worked on various informatics problems, which eventually led me into machine learning (ML), where we made predictive models for compound properties. We helped shorten and streamline the drug discovery process by examining aspects such as toxicity, efficacy and developing systems for virtual screening to minimize the amount of laboratory time and resources needed at pharmaceutical companies.”
Currently, Kerman works at Certara (Radnor, PA, USA), taking a slightly different focus on later-stage drug discovery — preclinical and beyond — that focuses heavily on the clinical trial stages using large language models (LLMs) and generative pre-trained transformers (GPTs) to help scientists, medical writers, and others make use of unstructured data. As an example, Kerman describes a researcher with a mountain of trial documents and reports from a lab or trial site. “They're able to use LLMs to process and get summarizations and information out of that data without having to scan each individual report,” he explains.
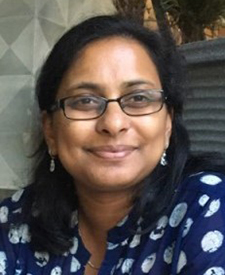
Reddy’s expertise is in ML, genomics and drug discovery. “I wanted to use data science and everything related to data analytics to further our understanding of diseases — to come up with targets and make an impact on patients,” she says.
As a computational biologist and data scientist with more than 12 years’ experience in the pharmaceutical industry and academia, Reddy previously worked at Novartis and Duke University Medical Center. She is currently co-founder of the data science consulting company, Vindhya Data Science (Research Triangle Park, NC, and Boston, MA, USA), which provides services related to bioinformatics, genomics, ML, AI, drug discovery, data engineering and epidemiology.
“We launched about three-and-a-half years ago. We are growing, and it's been a fun journey!” she adds. Reddy believes that the symposium offered in November is an opportunity to learn about cutting-edge data science and AI.
“I get lots of ideas at conferences — whether I see new techniques, similar problems and issues or new situations I haven’t encountered before — I am excited about what I learn,” she says. “I think that whether you need to use new technology in the near future or you're just curious about its capabilities, you can come and explore data sciences and AI and its role in the life sciences.”
Reddy eagerly anticipates the spatial biology and imaging session to be held at the symposium. “This is a modality of data that has been underutilized in the life sciences. There's so much information in an image, and usually it's just summarized with a few numbers,” Reddy comments. “With AI, we're able to capture a lot more information from it, and this can really benefit patients.”
She is also looking forward to the biomarker and foundation model sessions. “A classic example of a foundation model is ChatGPT, where the model learns not just one thing but a whole field. We've seen the power of ChatGPT, but you can imagine powerful foundation models for images — that can be the next revolution,” she comments.
Kerman expands on this idea: “Foundation models are the star child right now — that's what most people are talking about when they say they want to integrate AI into their laboratories. Researchers want to know — How can I use ChatGPT? Can I answer questions about my experiments? Can I get those sorts of insights without having to spend all the time reading all the minutiae of journal articles, research reports, and even ELNs?” he comments. He adds that exploring the session on how AI drives experimental inquiry and changes how researchers ask discovery questions will open new doors for life sciences professionals.
Reddy adds: “AI has the potential to enable all scientists to have conversations with data without worrying about coding or software expertise.”
“I think generating new ideas — such as those in the session about the next wave of intelligent systems — and hearing what other people are discussing has so much potential,” Kerman continues. “The field is so fast moving. You can’t predict what the next big idea is going to be. Conferences like these offer a mix of concepts and scenarios to foster ideas.”
Sidelines
Register Today for the SLAS 2024 Data Sciences and AI Symposium!
Explore the Data Science and AI Educational Track at SLAS2025
Investigate the SLAS Data Science and AI Topical Interest Group (TIG)